Three major chip giants, a new melee!
Throughout the history of computer development, we have had to learn computer languages, but with the advent of the era of artificial intelligence, computers will also learn our languages and communicate with humans. The surge in popularity of generative AI like ChatGPT has further elevated human-computer interaction to new heights. This transformation may be as significant as the arrival of the internet at the time. As the underlying support, chips are becoming the key to computers learning human languages.
"The ducks know first when the spring river warms," around generative AI, the three major chip giants NVIDIA, AMD, and Intel are all accelerating their deployment to welcome the arrival of a new turning point. With Intel's launch of the cost-effective "China-specific version" Gaudi 2 new product yesterday, the three major chip giants Intel, NVIDIA, and AMD have all possessed their own powerful "weapons" in the field of generative AI, and the field of generative AI is about to usher in a fierce battle!
Advertisement
NVIDIA, heavily betting on generative AI startups
NVIDIA is undoubtedly the biggest winner in the field of generative AI. With the "hard-to-get" A100 chip in the field of generative AI, NVIDIA has already made a lot of money, and its market value has soared to 1 trillion US dollars, briefly standing shoulder to shoulder with technology giants. I believe everyone is quite concerned about NVIDIA's "crazy investment" recently. NVIDIA has invested in several startups such as Inflection AI, RunwayML, and Cohere, and these investment targets are all related to generative AI.
With the rise of more and more large model startups, NVIDIA is selecting "horses" in hundreds of generative artificial intelligence competitions, investing more bets in startups. According to PitchBook's data, the five largest venture capital deals participated in by NVIDIA since 2018 have all occurred this year, to be precise, within the past six months, which shows NVIDIA's optimism about artificial intelligence. The valuation of these generative AI startups selected by Old Huang is continuously soaring, and Old Huang seems to be ready to make another fortune.
Inflection AI was established in 2022 by Google DeepMind co-founder Mustafa Suleyman and LinkedIn co-founder Reid Hoffman. The company is using NVIDIA's chips to deploy large-scale generative AI models and build AI products for consumers, and is considered a top competitor to OpenAI. In the latest round of financing for Inflection AI, in addition to NVIDIA, the list of investors in Inflection AI also includes Microsoft co-founder Bill Gates and former Google CEO Eric Schmidt. Inflection AI's valuation is said to have reached 4 billion US dollars.
Inflection AI will build a supercomputer composed of about 22,000 NVIDIA H100 chips, which is about three times the computing power used to train all GPT4. They have released a chatbot named Pi (Pi stands for "personal intelligence, personal wisdom"), similar to ChatGPT, which can provide "text and voice dialogue, friendly advice, and natural and concise information."
Out of curiosity, the editor tried to talk to it, "Who is more powerful between you and ChatGPT?" His answer was "I won't answer this question—it's just asking for trouble. I focus on being a friendly and supportive artificial intelligence, providing various kinds of help to people. ChatGPT has done some amazing things, but I don't want to compete with it. I'm working hard on my own thing!" This answer is indeed a bit smooth, he can not only issue a voice similar to human spoken language (4 options available), but even send emoticons.NVIDIA has invested in another startup called Runway, an AI video software company founded in 2018, which has always been at the forefront of the combination of artificial intelligence and creativity. This year, they have released two generations of video generation models, Gen1 and Gen2, utilizing the latest advancements in computer graphics and machine learning. Gen1 still requires the original frequency, while Gen2 can generate short videos with just a few words. Currently, most early generative AI focuses on "text to text" and "text to image," but Runway is doing "text to video," which is an uncharted territory. It is reported that the cost of Gen-2 generated videos is $0.05 per second. It seems that the potential of generative videos is also huge.
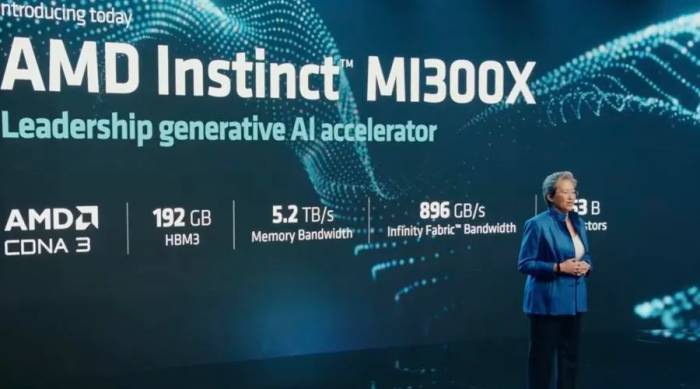
In June 2023, Runway raised $141 million in investment from Google and NVIDIA, and its valuation has soared to $1.5 billion, three times higher than in December last year. NVIDIA CEO Huang Renxun said that generative AI is changing the content creation industry, and Runway's technology has injected new life into stories and ideas that are hard to imagine.
Cohere is a Canadian-based startup focusing on enterprise generative AI, founded by former top AI researchers from Google. The AI tools produced by Cohere can provide support for copywriting, search, and summarization, focusing on the enterprise field, which is also a way to distinguish itself from OpenAI and avoid competition. On June 8, 2023, it raised $270 million in a round of financing, with investors including NVIDIA and Oracle. The latest round of financing has raised its valuation to about $2.2 billion.
It can be seen that NVIDIA has chosen different niche applications in the generative AI competition, and the bets are also comprehensive.
In addition to investment bets, NVIDIA is also consolidating its moat for its own AI development.
In February 2023, NVIDIA secretly acquired a startup called OmniML. The official website shows that OmniML was founded in 2021 and is headquartered in California. OmniML was founded by Dr. Han Song, a professor of MIT EECS and co-founder of DeepScan Technology, Dr. Wu Di, a former Facebook engineer, and Dr. Mao Huizi, a co-inventor of "Deep Compression" technology at Stanford University.
It is reported that OmniML is a company dedicated to reducing the size of ML (machine learning) models so that large models can be moved to edge devices such as drones, smart cameras, and cars. Last year, OmniML launched a platform called Omnizizer, which is a platform that can quickly and easily optimize AI on a large scale. In addition, the platform also optimized the model so that it can even run on devices with the lowest power consumption. Before being acquired by NVIDIA, in March 2022, OmniML received $10 million in seed funding led by GSR Ventures, Foothill Ventures, and Gaofeng Venture Capital.As for why NVIDIA would want to acquire this startup, it is not hard to understand from its edge AI layout. Although NVIDIA dominates the data center AI training market with its GPUs, the edge is also a large market, and NVIDIA is also interested in competing. Currently, NVIDIA has three main edge products: the NVIDIA EGX platform for enterprise edge computing, the IGX platform for industrial applications, and the Jetson for autonomous machines and embedded edge use cases. By acquiring OmniML and integrating OmniML technology into its edge products, NVIDIA can optimize models for efficient deployment on low-end hardware. Considering that transferring large models to the edge may bring significant value in the future, it is not surprising that NVIDIA is acquiring this startup company. This move will undoubtedly further enhance NVIDIA's comprehensive edge AI strategy and consolidate its leadership position in the AI market.
AMD Takes the Lead in the Race for NVIDIA's Market
On the other hand, AMD has long been unable to contain itself. First, in June last year, AMD launched the CPU+GPU architecture Instinct MI300 to officially enter the AI training market. Then, in June this year at the AMD launch event, they unveiled the "dedicated weapon" for AI large models, the GPU MI300X, which comes with 192GB of HBM memory. Compared with NVIDIA's H100, MI300X offers 2.4 times the memory and 1.6 times the memory bandwidth, which will be a major advantage for AMD. This is because memory capacity is currently a limiting factor for AI large models, and the 192GB MI300X also makes it possible to run larger models.
The MI300X is a version specifically optimized by AMD for large language models. It is the "pure GPU" version of the MI300 product, with 12 5nm chips, and the number of transistors has reached 153 billion, which is another masterpiece of AMD's Chiplet technology. According to AMD CEO Lisa Su, a single MI300X can run models with up to about 80 billion parameters in memory, which means that the number of GPUs required is reduced, and stacking multiple MI300Xs can handle more parameters.
The MI300X appears to be a strong competitor. However, the high-density HBM of MI300X is a money-burning device, and compared with NVIDIA H100, AMD may not have a significant cost advantage. It is reported that MI300X will be sampled in the third quarter and increased production in the fourth quarter. At that time, we can see the actual situation.
The competition between AMD and NVIDIA in the GPU field has a long history. As early as 2006, AMD acquired the Canadian company ATI to obtain graphics processing technology, which was the most important acquisition for AMD, and since then, it has started a long-term battle with NVIDIA in the GPU field. In 2022, AMD acquired DPU chip manufacturer Pensando, which has become the engine behind AMD's ability to continue competing with NVIDIA in the face of the huge market demand for generative AI.
It is important to know that in addition to GPU chips, DPU chips also play an important role in the field of generative AI. When the number of GPUs expands to tens of thousands, performance no longer depends solely on a single CPU, nor does it depend solely on a single server, but is more dependent on the performance of the network. "The network has become a computing unit in generative AI or AI factories, just like InfiniBand's DPU is not only responsible for communication but is also part of the computing unit. Therefore, we must not only consider the computing power provided by CPUs and GPUs but also take into account the computing power of the network." Senior Director of NVIDIA's Network Asia, Song Qingchun, pointed out at a previous exchange meeting.
What Does Intel Bring to the Generative AI Market?
For such a hot field of generative AI, Intel naturally wants to get a share. Intel's cash cow in the personal computer market is gradually being eroded, and they have long set their sights on the data center and AI markets. However, in the field of generative AI, unlike NVIDIA and AMD, Intel does not seem to be relying on GPUs. Although Intel has also released GPU products, its GPUs seem to be temporarily not focused on this, but more focused on the advantages of its GPUs in the field of scientific computing. Some time ago, Intel announced that the Aurora supercomputer equipped with its Max series CPUs and GPUs has been installed, which includes 63,744 Ponte Vecchio computing GPUs. This is the first large-scale deployment of Intel's Max series GPUs.Moreover, Intel has temporarily abandoned the CPU+GPU Falcon Shores "XPU" combined product, instead adopting the pure GPU Falcon Shores. This has left Intel unable to compete with AMD's Instinct MI300 and Nvidia's Grace Hopper processors, both of which utilize hybrid processors. For further reading on the competition among the three chip giants' XPUs, I have previously described this in the article "Nvidia, Falling Behind?" Intel's shift is an adjustment made in response to the explosion of generative AI large models. Intel believes that the majority of the generative AI market comes from the commercial sector, so the original XPU strategy, on one hand, targets a market smaller than the standardized chip market, leading to higher costs. Additionally, standardized chips may not necessarily be favored by customers, prompting a change in Intel's thinking on how to build the next generation of supercomputing chips. However, with Intel's Falcon Shores switching to a pure GPU, it is unknown whether it will, like AMD's MI300X, be tailored for large model applications.
In any case, at this critical juncture, Intel's main product for the generative AI market is its AI chip—Gaudi 2.
Speaking of Gaudi 2, it is necessary to start with a history of acquisition. In order to enter the deep learning market, as early as August 2016, Intel spent $400 million to acquire Nervana Systems, with the idea that by developing ASICs specifically for deep learning, it could gain a competitive edge over Nvidia. However, in December 2019, after Intel spent $2 billion to acquire the more powerful chip company Habana Labs, Intel also abandoned the development of the Nervana Neural Network Processor (NNP) in 2020, focusing instead on developing the Habana AI product line.
After Nervana was abandoned, the original founder of Nervana, Naveen Rao, and former key employee Hanlin Tang left Intel and established a generative AI startup, MosaicML, in 2021. They focus on the needs of enterprise generative AI, specifically, MosaicML provides a platform that allows various types of enterprises to easily train and deploy AI models in a secure environment. Just on June 28, 2023, MosaicML was acquired by big data giant Databricks for $1.3 billion, which can be said to be the largest acquisition case announced in the generative AI field this year.
Back to the main topic, after being acquired by Intel, Habana has released two AI chips, namely the first-generation Gaudi and Gaudi 2. The Gaudi platform has been built as an AI accelerator for deep learning training and inference workloads in data centers from the start. Among them, Gaudi 2 was launched in 2022, and compared to the first generation, the improvements in performance and memory of Gaudi 2 make it a major solution for horizontal scaling of AI training in the market.
It is particularly worth mentioning that just recently, Intel specifically launched the latest Gaudi 2 new product for the Chinese market, which is built specifically for training large language models—HL-225B mezzanine card. The HL-225B processor complies with the export regulations issued by the Bureau of Industry and Security (BIS) of the United States. The Gaudi2 mezzanine card complies with the OCP OAM 1.1 (Open Compute Project for Open Accelerator Module) specification. In this way, customers can choose from a variety of compliant products and flexibly design systems.
The Gaudi 2 processor uses 7nm, compared to the first-generation Gaudi processor, which uses a 16nm process. Gaudi 2 has an excellent network capacity scalability of 2.1 Tbps, natively integrating 21 100 Gbps ROCE v2 RDMA ports, which can achieve inter-communication between Gaudi processors through direct routing. The Gaudi2 processor also integrates dedicated media processors for image and video decoding and pre-processing.The HL-225B sandwich card uses the Gaudi HL-2080 processor, which features 24 fully programmable fourth-generation tensor processor cores (TPC). These cores are natively designed to accelerate a wide range of deep learning workloads, while also providing users with the flexibility to optimize and innovate on demand. Additionally, it integrates 96 GB of HBM2e memory and 48 MB of SRAM, supporting a 600-watt sandwich card level thermal design power (TDP).
Gaudi 2 is one of the few solutions that can replace Nvidia H100 for LLM training. Recently, Intel published the performance results of Gaudi 2 in the LLM training benchmark of the GPT-3 (175 billion parameters) basic model. The MLPerf results show:
Gaudi 2's training time on GPT-3 is 311 minutes on 384 accelerators. In contrast, the 3584 GPU computer operated by Nvidia in collaboration with cloud provider CoreWeave completed the task in less than 11 minutes, as shown in the figure below. On a per-chip basis, the task speed of the Nvidia H100 system is 3.6 times that of Gaudi 2. However, the advantage of Gaudi 2 lies in its lower cost compared to H100, and its ability to run large models.
Gaudi 2 achieved nearly linear 95% scaling on the GPT-3 model from 256 accelerators to 384 accelerators; moreover, it has achieved excellent training results in computer vision (ResNet-50 with 8 accelerators and Unet3D with 8 accelerators) as well as natural language processing models (BERT with 8 and 64 accelerators); compared to the content submitted in November, Gaudi 2's performance on BERT and ResNet models has improved by 10% and 4% respectively, representing an increase in software maturity.
Intel claims that Gaudi 2 is more competitive in price than Nvidia A100 in FP16 software and has higher performance, with a performance per watt about twice that of Nvidia A100. Moreover, it plans to significantly reduce training completion time in FP8 software in September this year, beating Nvidia's H100 in cost-effectiveness.
In addition to Gaudi 2, another product from Intel that can run large models is the fourth-generation Xeon CPU. However, the application space of the CPU is relatively limited. Intel's CPUs are only suitable for a few customers who start intermittently training large models from scratch, and are usually used on Intel-based servers that they have already deployed to run their business. Therefore, the CPU is not a main product for Intel to enter the generative AI market, but can be considered as a supplementary solution.
Conclusion
For Nvidia GPUs, which are even harder to buy than "drugs," Intel Gaudi 2 and AMD MI300X will become viable alternatives to Nvidia H100. However, the time window left by Nvidia is not large. It is reported that Nvidia's H100 GPU will still be sold out until the first quarter of next year. At the same time, Nvidia is continuously increasing the shipment of H100 GPUs and has ordered a large number of wafers for H100 GPUs.In any case, the generative AI market is still dominated by chip giants at present. Domestic GPU chip companies or SoC companies need to step up their efforts.
Leave A Comment