This chip hides the future of AI!
At the Computex in late May, Nvidia unveiled its new-generation supercomputer, the DGX GH200. According to Nvidia's official materials, the DGX GH200 supercomputer will be based on its Grace Hopper superchip. The DGX GH200 can contain up to 256 Grace Hopper superchips, providing up to 1 EFLOPS of AI computing power. Additionally, each DGX GH200 can provide up to 144TB of memory, with a bandwidth between the GPU and CPU reaching 900 GB/s. In terms of data interconnectivity, the DGX GH200 uses NVLink and employs Nvidia's self-developed NVSwitch network switch chip to meet the performance and scalability requirements of interconnectivity.
We can see that in the DGX GH200, the most critical chips, including CPUs/GPUs and data interconnectivity, are almost all self-developed by Nvidia. In contrast, the previous generation of supercomputers using the Hopper GPU, the DGX H100 SuperPOD, still uses Intel's Sapphire Rapids CPU. Moreover, due to Intel's CPU not providing an NVLink interface, it limits the scalability of its memory space— the memory of the previous generation DGX H100 SuperPOD is 20TB, while the DGX GH200 provides 144TB, directly more than 7 times. We will see in the following analysis that the expansion of memory space is one of the most critical indicators of current supercomputers, and after years of effort in chip architecture, Nvidia finally has the opportunity to use self-developed chips to achieve such a supercomputer with an astonishing memory space.
Advertisement
The DGX GH200 supercomputer is mainly aimed at ultra-high-performance artificial intelligence computing. According to current information from Nvidia, leading companies in the field of artificial intelligence such as Google and Microsoft will become the first customers of the DGX GH200.
The importance of supercomputers is highlighted in the era of large model artificial intelligence.
Currently, artificial intelligence has entered the next era dominated by large models. Large language models represented by ChatGPT can learn from massive amounts of corpus data and possess unprecedented capabilities. However, these large language models also have a considerable number of parameters. For example, according to existing information, OpenAI's GPT4 has about 1T of parameters. In addition to natural language processing, large model AI models are also widely used in recommendation systems and other fields. With the further development of these large models, it is expected that the number of parameters in large models will soon enter the 1T to 10T range.
Obviously, such models cannot be trained on a single conventional server because the memory of a single server is not enough to support the training/inference tasks of these large models. Therefore, in the field of artificial intelligence, the common practice is to distribute these large models (sharding) across multiple servers for training and inference.
For example, a large model can be distributed across 32 servers for training, where each server has its own independent memory space and is responsible for executing some layers of the large model's neural network (to ensure that the memory of each server is sufficient to accommodate the corresponding computing tasks). Then, after each server completes the calculation, the results are merged through the network to become the final result.
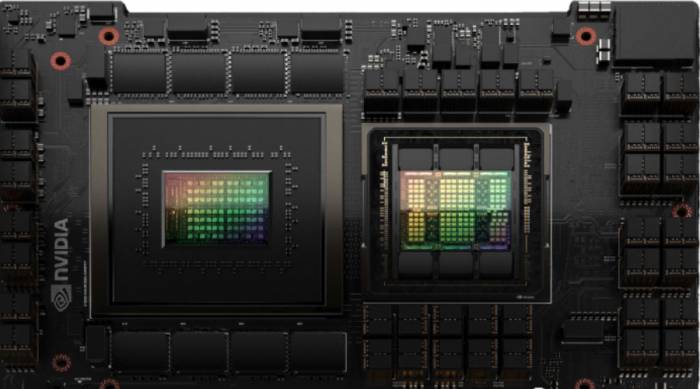
This conventional distributed computing approach can theoretically support infinitely large models as long as the model can be divided into sufficiently fine granularity to fit a single server. However, there is an obvious system bottleneck in this approach, which is that in such cloud-based distributed computing, each server is usually connected by a network (Ethernet or higher-speed InfiniBand), so the result merging part often becomes the system performance bottleneck. This is because, in the merging step, the data from each server needs to be transmitted through the network, and obviously, the more servers there are in distributed computing, and the smaller the network bandwidth/greater the latency, the worse the overall performance will be.
In this context, supercomputers have the opportunity to become an important computing paradigm in the era of large models. Slightly different from distributed computing, supercomputers emphasize concentrating high-performance computing units as much as possible and connecting them with short-distance ultra-high bandwidth/ultra-low latency data interconnects. Since the data interconnect performance between these computing units is much higher than using long-distance Ethernet/InfiniBand, the overall performance is not too limited by the data interconnect bandwidth. Compared with traditional distributed computing, using supercomputers can achieve higher actual computing power under the same peak computing power.
In the paradigm of the previous generation of AI computing (represented by ResNet-50 in the field of computer vision), the parameter amount of each model is about 100M, and there is no problem for each server to accommodate the model, so there is usually no issue of model sharding in the training process, and data interconnect is not the bottleneck of overall performance, so supercomputers did not receive so much attention. In the era of large models, since the model size has exceeded the limit that each server can accommodate, if high-performance training and inference are needed, supercomputers like the DGX GH200 have become a very good choice. Moreover, as the model parameter amount increases, more and more requirements are put forward for the memory capacity of supercomputers. Nvidia also released a comparison of the performance of DGX GH200 and the previous generation DGX H100. We can see that under the same number of GPUs, for large model applications, DGX GH200 with more memory and using NVLink has several times the performance of DGX H100 with less memory and using InfiniBand.Grace-Hopper Architecture Analysis
In the DGX GH200, the Grace Hopper superchip is utilized, with up to 256 Grace Hopper superchips capable of being installed in each DGX GH200.
What is the Grace Hopper superchip? According to the white paper released by Nvidia, Hopper is the latest GPU based on the Hopper architecture from Nvidia (i.e., the H100 series), and Grace is a high-performance CPU based on the ARM architecture developed by Nvidia itself. In terms of specifications, the Grace Hopper superchip can include up to 72 CPU cores, and the CPU is connected to up to 512GB of memory through the LPDDR5X interface, with a memory bandwidth of 546 GB/s. On the GPU side, it is connected to up to 96GB of video memory through the HBM3 interface, with a bandwidth of up to 3TB/s. In addition to the CPU and GPU, another crucial component in the Grace Hopper superchip is the NVLINK Chip-2-Chip (C2C) high-performance interconnect interface. In the Grace Hopper superchip, the Grace CPU and Hopper GPU are connected through the NVLINK C2C, which can provide a data interconnect bandwidth of up to 900GB/s (seven times that of x16 PCIe Gen5). Moreover, since NVLINK C2C can provide a consistent memory interface, the data exchange between the GPU and CPU becomes more efficient. The GPU and CPU can share the same memory space, and system applications can only move the data needed by the GPU from the CPU's memory to the GPU, without having to copy the entire block of data.
Physically speaking, the CPU and GPU chips of Grace Hopper are still two separate chips, and the interconnection also uses traces on the PCB board; however, logically, since both the CPU and GPU can see the same memory space, they can be regarded as a whole.
Scalability was clearly a top priority in the design of the Grace Hopper superchip. Here, NVLink once again plays a crucial role: each Grace Hopper superchip can use an NVLink Switch to interconnect with other Grace Hopper superchips at an ultra-high bandwidth of 900GB/s, and such interconnections can support up to 256 Grace Hopper superchips to form a superchip pod - which is also the interconnection method used in the DGX GH200 released by Nvidia this time. In addition, the Grace Hopper superchip can also connect to InfiniBand through an interface with Nvidia Bluefield DPU, allowing the superchip pod to further expand to a larger scale through InfiniBand, thereby achieving higher performance computing.
Through the above analysis, we see that in the Grace Hopper superchip, Nvidia's NVLink series of ultra-high-performance data interconnects play a crucial role, providing up to 900GB/s of bandwidth and a consistent interface, achieving very strong scalability. The difference between the CPU in the Grace Hopper superchip and other high-performance server-side ARM CPUs may be the support for the NVLink interface, which has become the biggest highlight of the Grace Hopper superchip.
Looking forward to the future competitive landscape, Nvidia has declared its leading acceleration capabilities for the next generation of large model artificial intelligence with the DGX GH200 supercomputer, which has astonishing performance and memory capacity. At the same time, we also see that designs like the Grace Hopper superchip, which tightly couple the CPU and GPU (as well as other accelerators) and provide efficient support for large models through ultra-high-speed consistent memory interfaces, will become an important design paradigm in the field of artificial intelligence chips in the future.
At present, in the field of supporting the next generation of large models, Nvidia is undoubtedly the most advanced player in the chip industry, and the manufacturer that has the potential to compete with Nvidia in the future may be AMD. In fact, AMD's design philosophy in this field is very close to that of Nvidia. Nvidia has the Grace Hopper superchip, while AMD's related product is the CDNA3 APU. In the CDNA3 APU architecture, AMD integrates the CPU and GPU together through chiplets and uses consistent data interconnects to support a unified memory space. In AMD's latest MI300 product, each APU integrates 24 Zen 4 CPU cores and several GPUs using the CDNA3 architecture (specific data to be released), and is equipped with 128 GB of HBM3 memory.If we compare the designs of Nvidia and AMD, we can see that the idea of tightly coupling the CPU and GPU and using a unified memory space is completely consistent, but there are several key differences in the specific design:
Firstly, AMD uses advanced chip packaging technology to achieve the integration of the CPU and GPU, while Nvidia, confident in its NVLink technology, uses traditional PCBs to integrate the CPU and GPU. However, this may change in the future; as the data interconnect bandwidth of PCBs approaches its limit, it is expected that Nvidia will also increasingly use advanced packaging technology for interconnection in the future.
Secondly, Nvidia is more aggressive in the field of memory space and scalability. The memory of each Grace Hopper superchip can reach 600GB, and through the NVLink Switch, it can even achieve a memory space of up to 144TB; in contrast, the memory space of AMD's CDNA3 APU is only 128 GB. Here, we see that Nvidia's long-term investment in the field of consistent data interconnect has obviously achieved very good results. In the future, in the field of large models, such ultra-high scalability data interconnect for expanding memory space is expected to become a key technology, and in this regard, AMD also needs to continue to invest to catch up with Nvidia's leading position.
Leave A Comment